Digital Rail are working on a project that seeks to use advanced Natural Language Processing (NLP) to identify non-compliance against railway standards during the development of railway system. Non-compliance will cause a lack of resilience which will be linked to likely train service delays and the associated carbon toll. The toll is caused by having to lay on additional rail services, buses, maintenance, and so on. The carbon toll of a system will therefore be known during the design phase which will be major step forward in understanding how clean our railways are likely to be.
It has been shown that modern electric railway transportation is environmentally friendly when compared to normal occupancy car transportation and carbon usage in the railway is mainly in operations. For a given concept of operation, the environmental impact of the system and the resilience are ”baked-in” in the downward part of the V lifecycle as shown in the Figure 1 below.
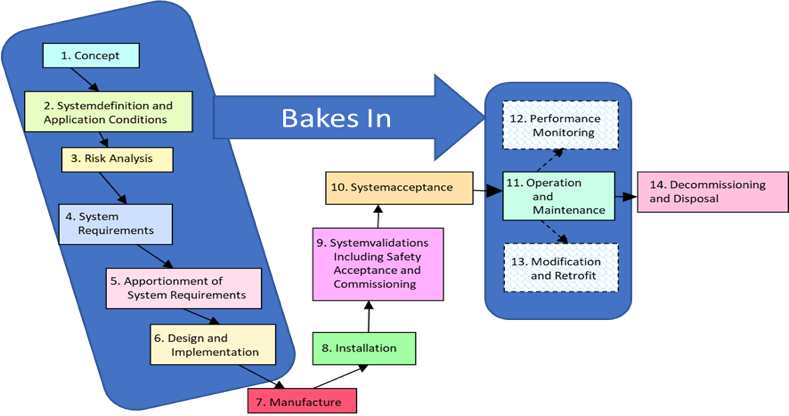
Figure 1. Development V Lifecycle
This part of the V Lifecycle includes requirements management and risk assessment (for operations) that we will monitor via a machine learning approach using NLP. The baseline is compliance with the standard in terms of resilience and any non-compliances will be identified by the NLP tool acting as a watchdog and identifying low quality activities. Risk assessment will identify events caused by lack of resilience (mainly safety and reliability) in operations and establish a relationship that will be mapped to carbon via a consequence model as depicted in Figure 2.

Figure 2. Linkage between Resilience and Environmental Impact
NLP is a technique that is not widely used in the rail industry and there is an opportunity to apply it to the critical systems engineering areas such as requirements, hazard log development and management. NLP is being applied more widely in other domains such as social media and advertising.
The environmental impact that is caused by lack of resilience includes: laying on additional train services, bussing, maintenance etc. This does not included the wider consequence to the public of lost working hours. Environmental Life Cycle Analysis (ELCA) during other phases is not in our scope.
The output of the project will be prototype NLP application programming interface (API) tools that can be plugged into a lifecycle management tool as depicted in Figure 3 below. This will enable resilience to be enhanced and the carbon impact to be understood more fully during railway operations.
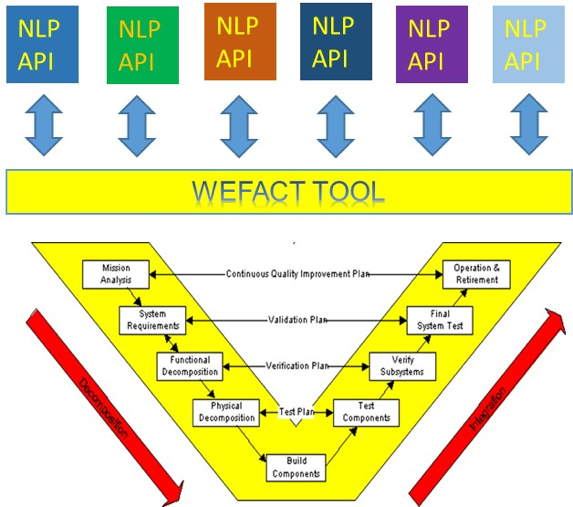
Figure 3. Intelligent High Resilience Low Carbon System Development Model
It has been shown that modern electric railway transportation is environmentally friendly when compared to normal occupancy car transportation. It has also been shown that the most of the environmental impact and carbon usage of a rail system is during operations [2]. Our tools will track standard compliance that ensures the resilience of the rail system. and understand how any lack of resilience will impact carbon expenditure by linking the two with an environmental model based upon service outages.
The environmental impact that is caused by lack of resilience includes: laying on additional train services, bussing, extra maintenance activities include car, van, plant usage, having to have redundant assets, passengers using other means, and the eventual decline of rail. This does not included the wider consequence to the public of lost working hours.